
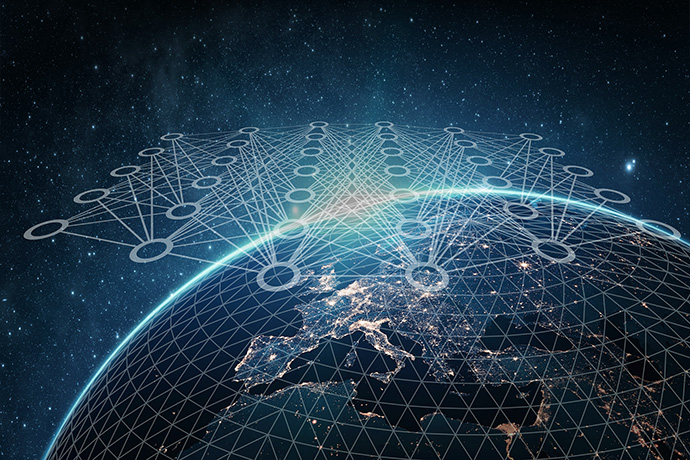
Almost 400 researchers from across the world joined the first ECMWF–ESA Workshop on Machine Learning for Earth System Observation and Prediction (ESOP), which was held online from 5 to 8 October.
The workshop brought together experts from a variety of diverse backgrounds to survey the state of the uptake of machine and deep learning (ML/DL) methodologies in the ESOP community, evaluate their expected impacts and build a consensus view on the best way to realise the untapped potential of ML/DL in this field.
Machine and deep learning techniques have made remarkable advances in recent years in a large and ever-growing number of disparate application areas, e.g. natural language processing, computer vision, autonomous vehicles, healthcare, finance and many others. These advances have been driven by the huge increase in available data, the increase in computing power and the emergence of more effective and efficient algorithms.
The Earth system sciences have arguably been latecomers to the ML/DL party, but interest is rapidly growing, as confirmed by the large number of participants in this workshop. Innovative applications of ML/DL tools are becoming increasingly common in ESOP. Introductory talks by David Gagne (NCAR) and Alan Geer (ECMWF) provided high-level overviews of the current status of ML/DL in the Earth sciences and its connections to more traditional methodologies.
One application of machine learning is in the study of extreme weather events such as flooding, tropical cyclones and drought. (Image: © nullplus / iStock / Getty Images Plus)
The workshop sessions covered the main building blocks of the numerical weather prediction (NWP)/climate prediction workflow (observations, data assimilation, models, ensembles and product development).
ML for Earth observations
Several speakers focused on the potential of ML/DL to improve the exploitation of available and future observations of the Earth system.
In areas of Earth observation based on image processing, ML/DL technologies are well established thanks to the application of existing network designs and strategies from the wider community. A number of talks indicated the great potential of ML/DL in providing insights from an increasingly vast stream of data from satellites: understanding what happens on Earth’s surface, analysing the dynamics, enhancing the capability of sensors and of the observations, etc.
In areas like nowcasting and NWP, Earth system observations can be more difficult to use – they are sparse, affected by uncertainties and made using indirect methods, often with substantial nonlinearities. There are, however, ways to address some of these problems, including using deep learning tools to emulate satellite observation operators, and even as a replacement for the whole process of data assimilation, and the research in this area is very active.
Pierre-Philippe Mathieu (ESA) said that machine learning offers a unique tool to transform petabytes of satellite data into actionable information.
ML for data assimilation
Participants identified three types of connections between ML/DL and the established methodologies in data assimilation (DA).
In the first, ML mimics, and possibly improves upon one piece of the DA algorithm, for instance the adjoint or the observation operator. In the second, DA and ML are two key ingredients of a hybrid algorithm and exchange information; for instance, DA is used to estimate a state trajectory while ML estimates the model or part of the model (i.e. model error). In the third type, ML and DA are unified under a consistent Bayesian framework, a natural extension of the classical Bayesian approach adopted in DA.
Marc Bocquet (Ecole des Ponts ParisTech), left, gave a presentation on using machine learning and data assimilation to learn both dynamics and state, in a session chaired by Alan Geer (ECMWF), right, on machine learning for data assimilation.
Massimo Bonavita (ECMWF) pointed out that, from a methodological point of view, ML/DL can be viewed as a particularisation of data assimilation to the task of estimating the underlying model dynamics and that some current operational data assimilation algorithms like weak-constraint 4D-Var already perform a form of online machine learning of the model errors.
This thought-provoking point leads to the question of how to further integrate ML/DL tools into state-of-the-art DA systems to improve both the estimation of the Earth system state and at the same time our modelling capabilities. Several key challenges have been identified, such as the identification of the optimal latent/state/control space to be used by both DA and ML routines (Rossella Arcucci, Imperial College), and the interfacing and inter-operability of different programming languages and libraries used in the ML and DA domains (Peter Houtekamer, Environment Canada).
ML for product development
Machine and deep learning can be used for extracting more information from the NWP workflow through the development of tailored, custom-oriented products. Applications of ML discussed at the workshop included the automatic detection of weather objects such as weather fronts and tropical cyclones, the downscaling of low-resolution forecasts and the production of weather products tailored for the renewable energy industry.
Another promising application area which was widely covered in both the oral and the poster sessions was the post-processing of ensemble forecasts. ML/DL was shown to be able to provide a flexible, data-driven modelling of nonlinear relations between predictors and the distribution parameters of the predictands, with results already competitive with state-of-the-art approaches.
Nikoli Dryden (ETH Zurich), right, presented results that show complex deep neural networks are useful for post-processing numerical weather prediction forecasts.
ML for weather and climate models
Participants also considered what ML/DL can do to improve weather and climate models. A promising application is in the emulation of model components, such as the radiation or gravity wave-drag parameterization scheme, in order to exploit the computational efficiency of ML/DL technologies and obtain speed-up and energy efficiency gains.
However, some speakers also showed that ML/DL has the potential for the development of new models (or model components) that may become competitive with existing conventional prediction models, in particular for nowcasting applications and seasonal predictions.
A hot topic in the application of ML/DL in NWP and climate is the use of physics-informed, interpretable and trustworthy ML methods. Imme Ebert-Uphoff (Colorado State University) and Vipin Kumar (University of Minnesota) explained that such methods would allow our understanding of the Earth system to be leveraged to develop customised ML tools that fit the needs of the community, instead of using black/grey-box ML tools whose results are difficult to interpret and for which it will be difficult to convince domain scientists about their trustworthiness. Furthermore, several approaches to learn a representation of model error and model uncertainty via ML/DL were discussed.
Outcomes
The oral and poster presentations sparked interest and lively discussions among the participants during four working groups covering the main areas of the application of ML/DL to NWP and climate. The main findings of the working groups were discussed in the final plenary session.
The general conclusion was that it was apparent that the field is in rapid development and there is huge interest in the community. It was also felt that progress will be fast as domain scientists increase collaboration with ML/DL specialists.
Presentations and recordings, including the working group findings, are available on the workshop web page.
To check on the pace of progress and further build the community effort, ECMWF and ESA have provisionally scheduled a second, follow-on workshop for Q4 2021. Details will be announced in due course.
ECMWF extends its thanks to partners at ESA, Météo-France and École des Ponts ParisTech for their help in preparing this article.