
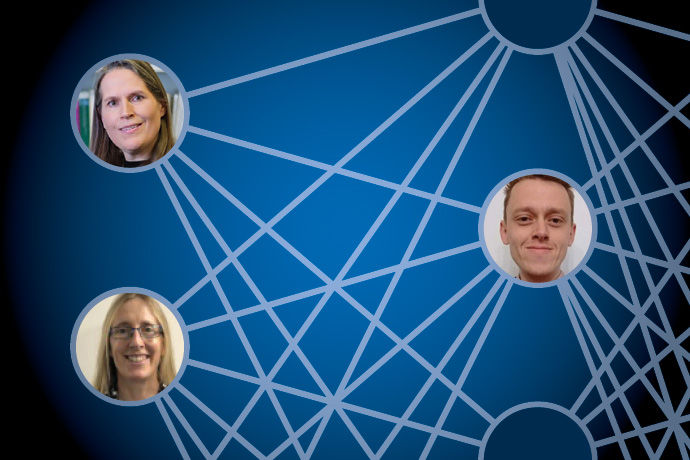
Christine Kitchen (top left), Victoria Bennett (bottom left) and Matthew Chantry (right) coordinate machine learning work at ECMWF.
Machine learning is becoming an increasingly important component of weather forecasting. At ECMWF, a team of three people has been established to coordinate related activities. They expect machine learning to continue to play a growing role in weather forecasting in the years to come.
The team has taken over from Peter Düben, who previously coordinated machine learning work at ECMWF. It includes members of three key Departments: Christine Kitchen, Deputy Director of Computing; Victoria Bennett, the Head of the User Services Section in the Forecasting Department; and Matthew Chantry, a scientist in the Research Department.
“Our role is to oversee machine learning activities and to drive forward strategic issues and progress,” says Victoria.
“We represent a range of skills and backgrounds as machine learning may be applied across different parts of the weather forecasting process,” Christine adds.
The only active machine learning scientist of the three is Matthew. “I’ve been working on machine learning for about five years, so the three of us have quite different sets of skills that we can bring to this role.”
What is machine learning?
Machine learning is a concept that lies between the more general idea of artificial intelligence and the narrower subfield of deep learning.

It refers to building models based on sample data to make predictions or take decisions. In numerical weather prediction, this contrasts with the traditional approach of using the laws of physics to make predictions.
This does not mean that machine learning would entail a loss of control over the weather prediction process.
“In applying machine learning, we don’t allow a computer to go away and learn with very little control over it. Rather, we have trained an algorithm to do a specific task. We know how it was trained to do something and what it was trained to do,” says Matthew.
Machine learning in weather forecasting
At ECMWF, machine learning has a role to play in many parts of the process of arriving at a numerical weather forecast and beyond.
Examples are the quality control of weather observations, the learning of model error within data assimilation, and the emulation of model components.
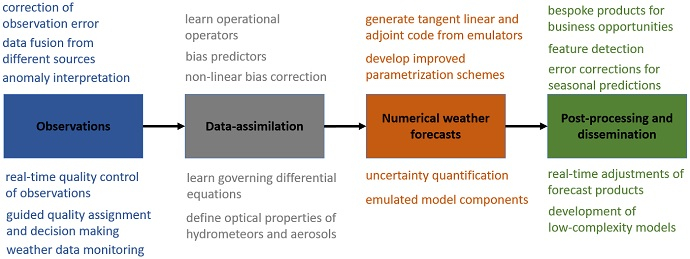
Potential areas of application for machine learning are spread over the entire numerical weather prediction workflow.
In the area of observations, for example, ECMWF has developed a machine learning tool for the detection and classification of observation anomalies.
This will improve the classification of events by severity and cause and will help to monitor satellite data from a growing number of platforms. It is still being tested, but it represents an application that is close to operational use.
Data assimilation is a field that has some similarities with machine learning, in that observational data are used to correct model output. In addition, in data assimilation attempts are being made to combine a physical model with machine learning methods to estimate and correct model error.
In the field of numerical weather forecasts, progress has been made in replacing traditional tools that are computationally very costly with machine learning tools, for example in the areas of the radiation scheme and gravity wave drag parametrization. But very high demands in terms of stability and accuracy have to be met before deploying these methods.
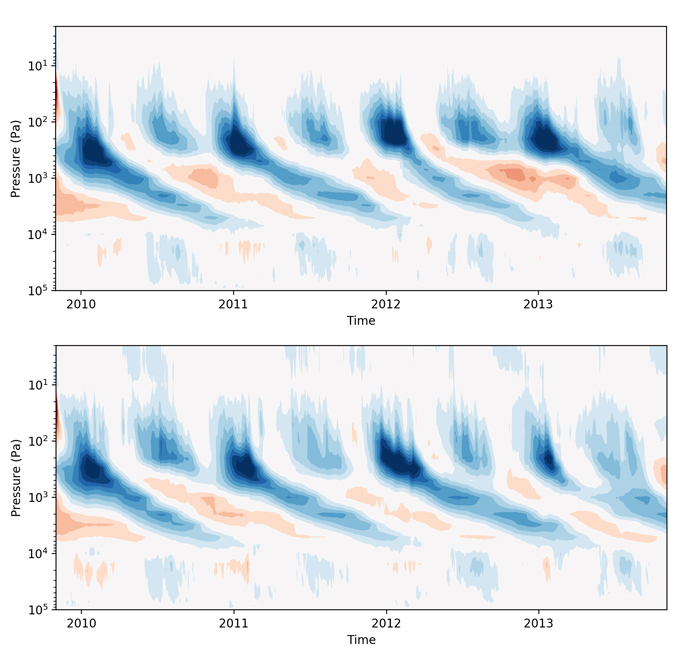
Forecasts with ECMWF’s Integrated Forecasting System of the zonal-mean zonal jet averaged between latitudes -5 to 5, depicting the quasi-biennial oscillation (QBO). Top: using an increased complexity version of the existing non-orographic gravity wave drag scheme. Bottom: using a neural network to emulate non-orographic gravity wave drag. Both forecasts capture the phases of the QBO and only diverge after significant simulation time.
The current growth in machine learning in the area of weather prediction is partly the result of more data being available.
“Some of the concepts behind machine learning have been around for a long time, but over the last few years the availability of data has witnessed rapid growth, and technology has evolved to the point of allowing some of the deeper levels of computation to be accomplished,” says Christine.
The growing role of machine learning in numerical weather and climate predictions is reflected in a Massive Open Online Course (MOOC) on the topic, which ECMWF is running until April this year.
What the future may hold
Opinions vary to what extent machine learning may replace more traditional methods in the future.
At a recent ECMWF–European Space Agency workshop on machine learning for Earth observation and prediction, some participants suggested that it will eventually replace today’s methods in numerical weather prediction.
This belief is fuelled by the availability of high-quality historical datasets, such as ECMWF’s ERA5 reanalysis of the global weather and climate. ERA5 covers many decades and has been produced using traditional methods.
Most of those taking part in the conference foresaw a more complementary co-existence of traditional and machine learning techniques in weather prediction: in aspects of data assimilation, forecasting and post-processing.
“Nevertheless, I wouldn’t rule out machine learning being a big component of the forecasting system in ten years’ time if progress continues at the current rate,” says Matthew.
He points out that the computational resources required to make a prediction using machine learning are much smaller than in traditional methods. This would make it possible, for example, to use a much bigger number of ensemble members than in current forecasts.
Meanwhile, ECMWF’s machine learning coordinators are keeping a close eye on developments both internally and externally.
“We aim to make sure the right kind of people are talking to each other internally, but I also see us as visible contact points for external parties in this field to establish connections to the Centre,” Victoria says. “We also try to ensure that more machine learning work can be carried through to operations.”